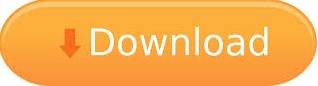

KEYSTROKES PER HOUR SERIES
The research used the dynamic interrelation between various different regions of interest (ROI) on the human body (face, body, arms, legs) and the time series based events related to the these ROIs. The actions of lifting objects and walking in the room, sitting in the room and neutral standing pose were used for testing the classification. This paper examines use of dynamic probabilistic networks (DPN) for human action recognition. The decision rule driven and activity templates method produced 64% recognition accuracy indicating that the method was feasible for recognizing human activities. This candidate frame was compared with the decision rule driven model to associate with an activity class label. The image data frames are down sampled using activity templates to a single candidate frame. The extracted shape is transformed to binary state using eigen space mapping and parametric canonical space transformation. The human shape is extracted using geometric model across multiple frames. This paper uses dynamic, temporal data to compare with decision rules and templates for activity recognition. There is additional information in the movement based features and temporal series of frames which can be leveraged to identify a certain action.
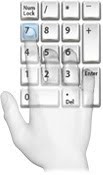
Several human activity recognition systems incorporate analysis of static features and posture based coordinates to detect activity. Human activity recognition has many real world applications such as surveillance, assistive robotics and simulation systems. The results showed improvement in emotion recognition accuracy (The precision increased by 3.28% and the recall rate by 3.17%) when the 3D geometric, kinematic, and human behavioral pattern-based features were combined for multimodal emotion recognition using supervised classification. The classifier was trained using 10-fold cross validation and support vector machine (SVM) to predict six basic emotions. The features from each modality and the behavioral pattern-based features (head shake, arm retraction, body forward movement depicting anger) were combined to train the multimodal classifier for the emotion recognition system. Human emotional behavior-based features were developed using inter-annotator agreement and commonly observed expressions, movements and postures associated to specific emotions. The 3D geometric and kinematic features were developed using raw feature data from the visual channel. Head, face, hand, body, and speech data were captured from 15 participants using an infrared sensor (Microsoft Kinect). This paper presents a multimodal emotion recognition method that uses a feature-level combination of three-dimensional (3D) geometric features (coordinates, distance and angle of joints), kinematic features such as velocity and displacement of joints, and features extracted from daily behavioral patterns such as frequency of head nod, hand wave, and body gestures that represent specific emotions.
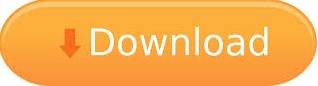